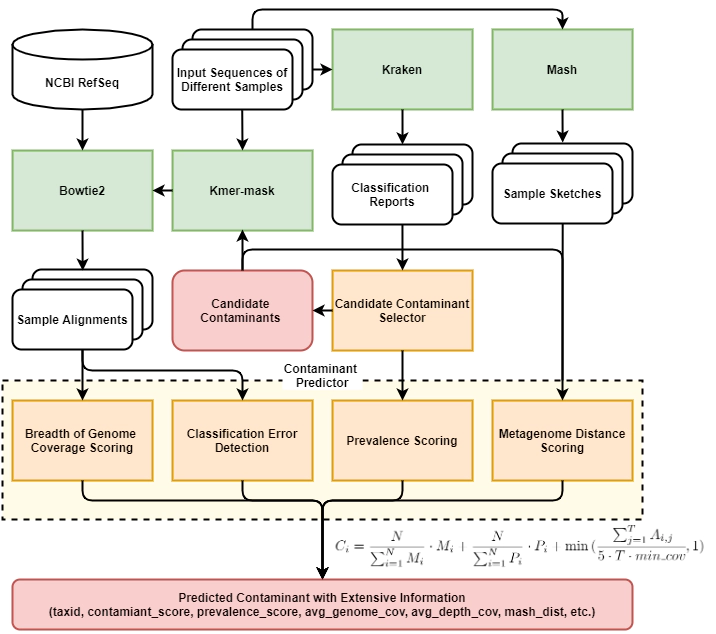
Background
Computational analysis of host-associated microbiomes has opened the door to numerous discoveries relevant to human health and disease. However, contaminant sequences in metagenomic samples can potentially impact the interpretation of findings reported in microbiome studies, especially in low biomass environments. Although this have been a known issue for some time, negative control data are often not available in public databases, making it nearly impossible to perform contamination removal on uploaded data.
Findings
Our hypothesis is that contamination from DNA extraction kits or sampling lab environments will leave taxonomic “bread crumbs” across multiple distinct sample types, allowing for the detection of microbial contaminants when negative controls are unavailable. To test this hypothesis we implemented Squeegee, a de novo contamination detection tool. We tested Squeegee on simulated and real low biomass metagenomic datasets. On the low biomass samples, we compared Squeegee predictions to experimental negative control data and show that Squeegee accurately recovers known contaminants. We also analyzed 749 metagenomic datasets from the Human Microbiome Project and identified likely previously unreported kit contamination. Collectively, our results highlight that Squeegee can identify microbial contaminants with high precision.
Conclusions
In summary, Squeegee is the first computational method for identifying potential microbial contaminants in the absence of environmental negative control samples. Squeegee is open-source and available at: https://gitlab.com/treangenlab/squeegee
Collaborators
- Dr. Kjersti Aagaard (BCM)
- Dr. Michael Jochum (BCM)