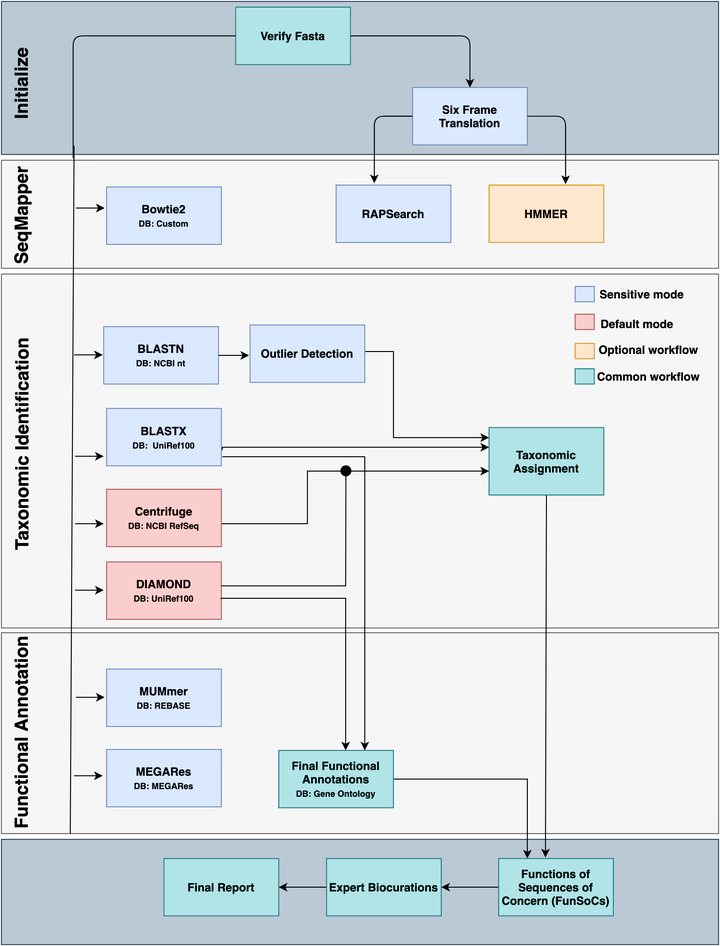
Background
The COVID-19 pandemic has emphasized the importance of detecting known and emerging pathogens from clinical and environmental samples. However, robust characterization of pathogenic sequences remains an open challenge. To this end, we developed SeqScreen, which can accurately characterize short nucleotide sequences using taxonomic and functional labels, and a customized set of curated Functions of Sequences of Concern (FunSoCs) specific to microbial pathogenesis. We show our ensemble machine learning model can label protein-coding sequences with FunSoCs with high recall and precision. SeqScreen is a step towards a novel paradigm of functionally informed pathogen characterization.
Collaborators
- Dr. Krista Ternus (Signature Science, LLC)
- Dr. Gene Godbold (Signature Science, LLC)
- Dr. Anthony Kappell (Signature Science, LLC)
- Dr. Madeline Diep (Fraunhofer USA Center Mid-Atlantic CMA)
- Dr. Daniel Nasko (UMD)
- Dr. Nidhi Shah (UMD)
- Dr. Mihai Pop (UMD)
- Dr. Santiago Segarra (Rice University)
Sequencing data
- Illumina